Review Article
Molecular Docking: A Paradigm Shift in Modern Drug Discovery Process
1045
Views & Citations45
Likes & Shares
More recently in computational chemistry drug discovery involves modern concept of molecular docking which is in-silico structure-based method. Utilization of time, money and human resources in effective manner is very much important nowadays, in this context molecular docking become a key tool for recent drug development with purpose of enhanced efficiency and research cost reduction. It is very important part of bioinformatics which deals with the interaction of protein and ligand molecule. Based upon the properties of ligand and target molecule, it predicts the three-dimensional structure of any complex. To optimize the conformational structures with the intention of possessing less binding free energy is the objective which is of utmost importance in molecular docking. Molecular modeling based on effective utilization of all the theoretical and computational techniques for imitate the behavior of molecules. The main aim of presenting this review is to summaries the whole docking methodology for the purpose of knowledge transfer. This review article presents the brief introduction of models of molecular docking, different approaches, scoring functions, software used in docking process and applications of molecular docking.
Keywords: Molecular modelling, Molecular docking, Approaches, Scoring functions, Software
INTRODUCTION
Nowadays, in computational chemistry, molecular modeling is a very important tool for drug discovery and design. It helps to understand the chemistry of any new molecule very effectively, but the need is the adequate knowledge of the available software and the appropriate interpretation of the results. To describe the use of computers to construct molecules and perform a variety of calculations on these molecules to predict their chemical characteristics and behavior, molecular modeling is applied. In general, the term computational chemistry is often used as a synonym for the term molecular modeling. But computational chemistry is a broader term, referring to any use of computers to study chemical systems. Some chemists often use the term computational quantum chemistry to refer to the use of computers to perform electronic structure calculations, where the electrons in a chemical system are calculated [1].
Molecular modeling comprises the concept of all theoretical method and computational techniques, so that it will be easy to model or mimic the behavior of molecules. To perform detailed and result oriented study molecular systems ranging from small chemical systems to large biological molecules and sets of materials such techniques are used in the fields of computational chemistry, computational biology, and materials science. By applying molecular modeling there is great reduction in the complexity of the system and allowing many more particles (atoms) to be considered during simulations which are the major benefit of molecular modelling [2]. In recent years, the search for new drugs has evolved from a trial-and-error process which is time consuming with the uncertainty of results to a sophisticated procedure that includes several computer-based approaches with accuracy in results. To discover new compounds of therapeutic relevance, structure-based design can be applied in which the structures of known target proteins are used. In structure-based drug design (SBDD) the main approaches can be utilized which are classified roughly into two categories: de novo design and docking [3] (Figure 1).
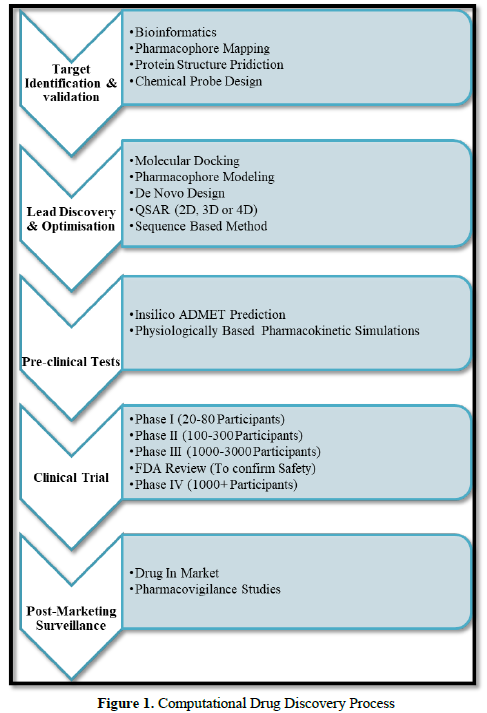
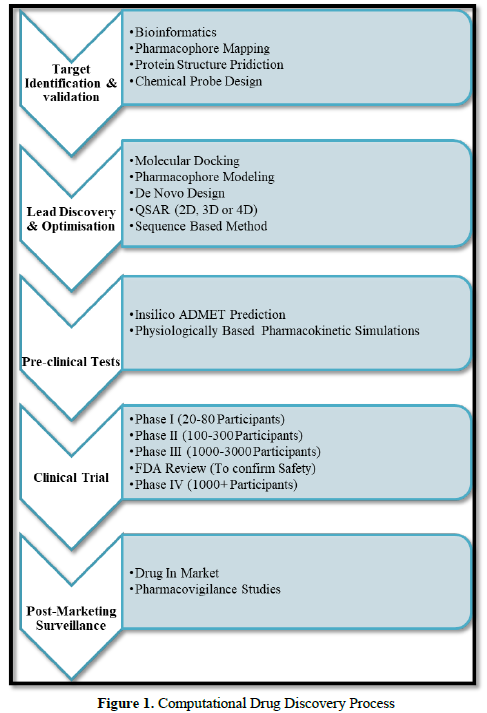
Molecular Docking
In the particular branch of molecular modeling, the molecular docking method is used for predicting the preferred orientation of one molecule with respect to another when linked to each other will constitute a stable complex. Adequate knowledge of the preferred orientation is very essential and, in turn, it can be useful to predict the strength of the association, stabilities, less is the energy more will be the stability or the binding affinity between two molecules using the scoring functions. The interaction between biologically important molecules such as carbohydrates, proteins, nucleic acids, and lipids plays an essential role in signal transduction. Furthermore, the type of signal produced can be affected by the relative orientation of the two interacting molecules. Therefore, molecular docking is very useful for result oriented prediction in both the strength and type of signal produced. Hence, molecular docking is effective tool of computational method utilize to predict the interaction of two molecules generating a stabilized binding model. Molecular docking is applied in many drugs discovery processes; previously docking is done between a small molecule and a macromolecule for example, protein-ligand docking only. But more recently, docking is also applied for macro molecules, for example protein-protein docking for prediction of the binding mode and stable orientation between two [4] (Figure 2).
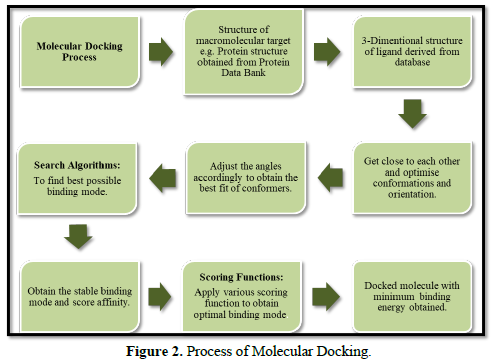
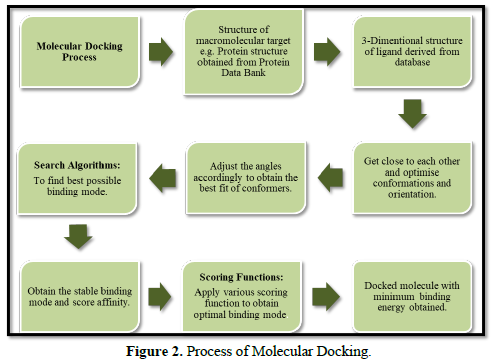
Molecular Docking Models
Molecular docking generally utilizes two basic models which are as follows:
- Lock and Key Model: This model mainly emphasizes on geometric complementarity. Rigid docking method is based on this model. Both receptor and ligand are fixed geometrically and binding occur only at a particular conformation just like ‘Key’ fits into the ‘Lock’.
- Induced fit Model: This type of model is widely used which utilizes geometric complementarity and the energy complementarity. Docking process is so flexible that receptor and ligand have to change their conformations to provide most stable in such a manner that minimizes the free energy [5].
Methods of Molecular Docking (Table 1)
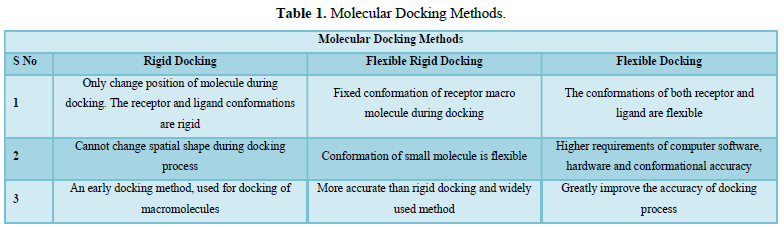
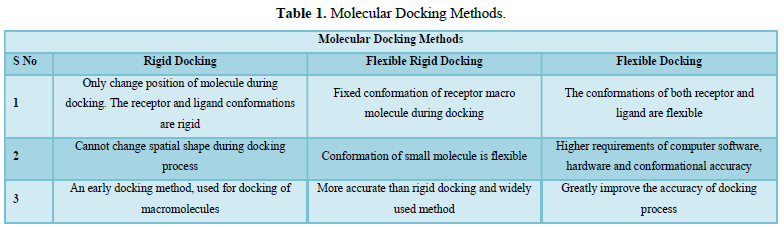
APPROACHES IN MOLECULAR DOCKING
Simulation Approach: This is much more complicated approach in docking process.
Method
- In this approach, there is a certain physical distance between the protein and the ligand molecule, and after a number of ‘movements’ in the conformational space, the ligand finds its position at the active site of the protein.
- Due to such moves, there will be incorporation of rigid body transformations (externally), such as translations and rotations, as well as internal changes in the ligand structure may occur (internally) such as, torsion angle rotations.
- In the ligand conformation space, each of these movements induces a “Total Energy” cost of the system and, therefore, after each movement the total energy of the system is calculated [6].
Advantages
- It is more compatible to incorporate ligand flexibility into its modeling while shape complementarily techniques have to incorporate some ingenious methods to incorporate flexibility in ligands.
- It is more real to evaluate the molecular recognition between flexible ligand and protein target.
Disadvantage
It takes longer to evaluate the optimal bonding pose as they need to explore a rather large energy landscape. However, grid-based techniques and rapid optimization methods have significantly improved these problems.
Docking can be between:
- Protein / ligand
- Protein / peptide
- Protein / protein
- Protein / nucleotide
SHAPE COMPLEMENTARITY APPROACH
Method
- Geometric matching/ shape complementarily methods describe the protein and ligand as a set of characteristics that make them dock able.
- These features may include molecular surface/ complementary surface descriptors.
- In geometric matching, solvent-accessible surface area expressed the molecular surface of receptor while matching surface description expressed the molecular surface of ligand.
- The shape matching between the two surfaces described by the complementarily.
- By utilizing this complementary pose (shape matching) of docking the target protein and the ligand molecules can easily be find out.
Advantages
- The geometric matching based approaches of molecular docking are typically fast and robust.
- Recent advancements allow these methods to also investigate ligand flexibility.
- Complementary methods can steadily scan through several thousand ligands in a matter of seconds and really assess if they are able to bind at the active site of the protein, and are generally scalable for even protein-protein interactions.
- For pharmacophore-based approaches, these methods are much more compatible also, because they utilize geometric descriptions of the ligands to find optimal binding [7-9].
Search Algorithms
Molecular Docking can be categories by their search algorithms, which are interpreted by a set of rules and parameters applied to predict the conformations. In docking method, flexibility of the ligand and/or the receptor docking algorithms can be classified in two main groups: rigid-body and flexible docking. The rigid-body docking method does not allow flexibility of neither ligand nor receptor considering essentially geometrical complementarities between two molecules but, limits the specificity and accuracy of results. These types of docking simulations were able to predict the correct position of ligand, while comparing the crystallographic structures [10-14]. ZDOCK can be used for all rigid-body docking simulations, and superposition of the best results, estimated using empirical scoring functions, against crystallographic structures generated RMSD lower than 1.0 Å. This method has been utilized as the fastest way to perform an initial screening of a small molecule database and also employed for virtual-screening initiatives. It has a relatively high accuracy, as compared to crystallographic structures.
Wherever, in flexible docking methods modifications in several possible conformations of ligand or receptor, as well as for both molecules at the same time, at a higher computational time cost can be considered. For applying a more specific refinement and lead optimization after initial rigid body docking procedure, flexible docking has been utilized. Various search algorithm method is described below which can be utilized for docking:
Fast Shape Matching (SM)
In this type of algorithms, the geometrical overlap between two the molecules take into consideration. Different algorithms are used in order to achieve different alignments between ligand and receptor. This approach can identify possible protein binding sites by means of a macromolecular surface search. Furthermore, specific SM algorithms establish possible conformations of the expected binding sites [15].
Incremental Construction (IC)
This method divides the ligand into fragments that are docked separately at the receptor site. On docking of the fragments, docked the parts are fused together. This fragmentation allows the algorithm to consider ligand flexibility. Initially, the docked rigid fragments function as "anchors" that are secondarily joined by flexible parts of the ligand that have rotatable linkages. In this way, the ligand gradually "builds" within the receptor binding site. It is also known as anchor and-grow method; IC algorithm has been employed in several docking programs such as DOCK, FLEXX etc. [16-18].
Monte Carlo (Mc) Simulations
MC simulations were originally introduced as an energy minimization procedure in molecular dynamics applications, such as those implemented in GROMACS and GROMOS [19-21] and have recently been adapted for flexible docking algorithms such as MCDOCK and ICM [22,23]. It is a good method to carry out analysis of bio molecular systems under different thermodynamic conditions.
Simulated Annealing (SA)
This method has various applications and has been subject of several studies, which includes conformational-analysis, protein structure prediction studies, and molecular docking search methods. By a specific kind of dynamic simulation, a bio molecular system is simulated. Each docking conformation is led to a simulation where the temperature gradually decreases over regular time intervals in each simulation cycle. It may give a higher accuracy result when compared with MC, since it considers more detailed the conformational state and flexibility of both protein and ligand in different thermodynamic states during an interval of time [24,25].
Nevertheless, SA docking may be more time consuming, since the annealing cycle must be repeated for each ligand placed inside receptor site. In order to minimize computational cost, filtering procedures may be carried out between ligand placement and SA cycles. SA may be combined with MC, as in Auto Dock, one of the most popular software packages for docking, that uses a Monte Carlo Simulated Annealing (MCSA) protocol, where random changes are made in ligand orientation inside protein binding site during each SA temperature cycle. The energy of current state is compared with previous state energy and the lowest energy is chosen to be compared with next state. Otherwise, the configuration is accepted or rejected basing on Boltzmann equation that follows: P = e (- ΔE/ kT)
Where P is the acceptance probability, ΔE is the difference in energy from the previous step, T is the absolute temperature in kelvin, and k is the Boltzmann constant.
Genetic Algorithm (GA)
In this algorithm, crossover is applied, which is a genetic operator that combines (pairs) two chromosomes (parents) to produce a new chromosome (offspring), if it takes the best characteristics of each parent then generated offspring may be better than both parents. This process that swaps large regions of the “parents,” is permitted in genetic algorithms. Many complex scoring functions are used in this process, taking into account a set of parameters, such as mutation rates, crossing rates, and the number of evolutionary rounds. This algorithm is also applied in the program DOCK, which is able to dock either whole ligand inside active site or a rigid fragment of the ligand [16].
“Lamarckian” GA (LGA) is also very much applicable in docking algorithms. The LGA switches between “genotypic space” and “phenotypic space.” In genotypic space mutation and crossover occur, while phenotypic space is described by the energy function to be optimized. After genotypic changes have been made to the population (global sampling) in phenotypic space, energy minimization (local sampling) is performed, which is conceptually similar to MC minimization. Phenotypic changes of energy minimization are mapped back to genes (changing the coordinates of the ligand on the chromosome) [26] One of the most popular molecular docking programs that use LGA is the program AUTODOCK [27].
Tabu Search (TS)
Tabu search (TS) is an iterative procedure designed for obtaining solution of optimization problems. It was developed and described by Glover and has been used to solve a large variety of hard optimization problems. This procedure can be defined as a Meta-Heuristic methodology that can move from a solution to another being able to save in memory the already visited solutions. Recently, the probabilistic heuristic algorithms have been given wider applications. The TS docking algorithm has demonstrated high accuracy, being able to avoid that the simulation which can trapped in local minima and avoiding visiting known minimum energy conformations. Currently, it has been utilized to predict the conformations of a test set of 50 binary complexes, presenting the RMSD below 1.5 Å [28].
Scoring Functions
Another major hurdle in docking is imperfection in scoring function. Scoring functions is another important parameter in docking which provide optimum conformation with minimum binding energy just like search algorithm; also, it should also be able to differentiate true binding modes from all the other parallel modes. A scoring function should have the properties of computationally economical, unfavorable for analyzing various binding modes. To assess optimum ligand affinity scoring functions make number of suggestions according to accuracy. The physical features i.e., electrostatic interactions and entropy are not considered in scoring schemes. So, the unavailability of appropriate scoring function, both in terms of speed and accuracy, is the major bottleneck in molecular docking programming [29].
The main objective of the scoring function is to describe the correct poses from incorrect poses, or binders from inactive compounds in an appropriate computation time. Moreover, the scoring functions are based on estimation of the binding affinity rather than calculation between the protein and the ligand and through these functions, adopting various hypotheses and simplifications. Scoring functions are classified as force-field-based, empirical, knowledge-based, and consensus scoring functions [30].
Force-field-based scoring functions [31-33] based on the calculation of the sum of the interaction energies of protein-ligand complex such as electrostatics and van der Waals (non-bonded) for the evaluation of the binding energy. By the use of Coulombic formulation, the electrostatic terms are calculated and by Lennard-Jones potential function van der waals terms are described. Utilizing different parameter sets for the Lennard-Jones potential which defines closeness of contact between protein and ligand atoms so that they can be acceptable. Generally, cut-off distance is used to tackle the non-bonded interactions because force-field-based scoring functions have the disadvantage of slow computational speed. Software programs, such as DOCK, GOLD and Auto Dock [16,26,34] are the examples of such scoring functions.
Empirical scoring functions [35-39] based on break down of binding energy into several energy components, such as hydrogen bond, ionic interaction, hydrophobic effect and binding entropy. Further multiplication of each component is done by a coefficient and then summation will give a final score. By the use of regression analysis, coefficients are obtained which then fitted to a test set of ligand-protein complexes with known binding affinities. For the analysis purpose, empirical scoring functions have relatively more simplified energy terms. Also, by using different software, each term in the empirical scoring functions can be treated differently, and the numbers of the terms included are also different. LUDI, Chem Score [40,41] are software’s derived for empirical scoring functions.
Knowledge-based scoring functions [42-47] based on statistical analysis of ligand-protein complexes crystal structures. The main concern is to obtain inter atomic contact frequencies and/or distances between the ligand and protein. This method is founded on the hypothesis that the more favorable the interaction is, more will be the frequency of occurrence. Further these frequency distributions are converted into pair wise atom-type potentials. The calculation of score is done by favoring preferred contacts and penalizing the repulsive interactions between each atom in the ligand and the protein within a given limit. The computational simplicity is the major advantage of this scoring function, which can be utilized to screen large libraries of compound databases. Some uncommon interactions like sulphur-aromatic or cation-π can also be model by this, which are often limited in empirical approaches. DrugScore, SMoG [48,49] and Bleep are examples of knowledge-based functions.
Consensus scoring [50] is a recently widely used method that combines various different scores to evaluate the docking conformation. A ligand conformation or a potential binder could be accepted when qualified well under several different scoring schemes. This scoring function substantially enhances enrichments (i.e., the % of strong binder among the high scoring ligands) in screening, and increase the prediction efficiency of bound conformations and poses [51]. Furthermore, the utility of consensus scoring decreases when the terms in different scoring functions are significantly correlated; CScore [52] which is the combination of various other scoring software; DOCK, ChemScore, PMF, GOLD, and FlexX is an example of consensus scoring function.
APPLICATIONS OF MOLECULAR DOCKING
In current scenario of computational chemistry, there are several applications of molecular docking due high demand of drug discovery with more accuracy in result prediction along with the requirement of optimum time duration and computationally economic too. Docking may be applied to:
- Hit Identification - Docking combined with a scoring function can be used to quickly detect large databases of potential drugs insilico to identify molecules that can favorably bind to the target protein of interest.
- Lead Optimization - it can be utilized to predict the best-defined location and in which relative conformation a ligand binds to a protein (binding mode or pose prediction). This information in turn can be used to design more powerful, selective and efficient analogs.
- Bioremediation - Protein ligand docking can also be used to predict pollutants and contaminants that can be degraded by enzymes.
- Estimation of the binding affinity
- Searching for lead structures for protein targets
- Comparing a set of inhibitors
- Estimating the influence of modifications in lead structures
- De Novo Ligand Design
- Design of targeted combinatorial libraries Predicting the molecule complex
- Understanding the binding mode / principle
- Optimizing lead structures
- Vaccines preparation by structural recognition
CONCLUSION
Molecular Docking is very safe, easy and efficient tool in computational chemistry helps in investigating, interpreting, explaining, identification and by using various scoring functions optimization of molecular properties can be done using three-dimensional structures. It attempts to predict the structure of the intermolecular complex formed with optimum stability between two or more constituent molecules and assess binding energy also provides the best conformational pose. These techniques greatly help to create libraries of compound within optimum computational time duration and are applied in the fields of computational chemistry, computational biology and materials science for studying molecular systems ranging from small chemical systems to large biological molecules. Most of the docking programs presently being used simulate the binding and their energies of a flexible ligand to a rigid biological receptor. This model does not reflect the actual physical process of binding and limits or in some cases even prevents the correct identification of potential drug candidates. But it is very remarkable development in the drug discovery process.
Software Used for Docking (Table 2)
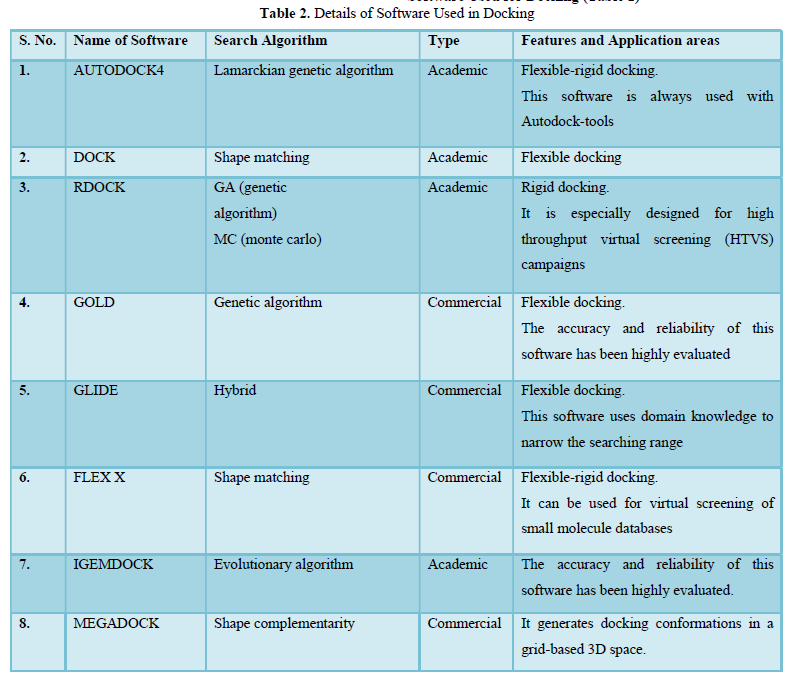
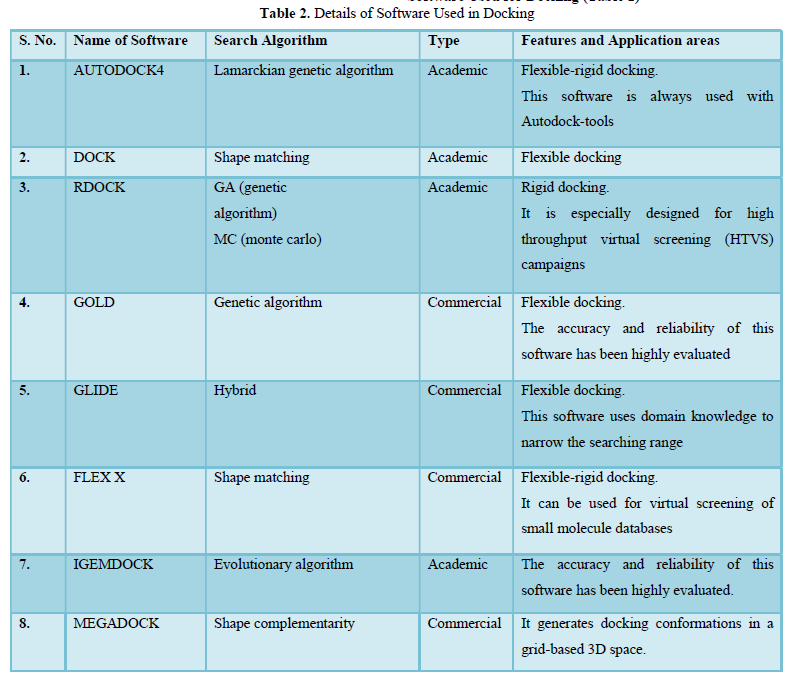
ACKNOWLEDGEMENT
Moral and Educational support provided by the Swami Vivekanand College of Pharmacy; Indore are gratefully acknowledged.
CONFLICT OF INTEREST
In this review article there is no conflict of interest.
- Nadendla RR (2004) Molecular modeling: A powerful tool for drug design and molecular docking. Resonance 9(5): 51-60.
- Mukesh B, Rakesh K (2011) Molecular docking: A review. Int J Res Ayurveda Pharm 2(6): 1746-1751.
- Claussen H, Buning C, Rarey M, Lengauer T (2001) FlexE: Efficient molecular docking considering protein structure variations. J Mol Biol 308(2): 377-395.
- Koshland DE (1963) Correlation of structure and function in enzyme action. Science 142: 1533-1541.
- Singh B, Mal G, Gautam SK, Mukesh M (2019) Computer-Aided Drug Discovery in Advances in Animal Biotechnology. Springer Cham 2019: 471-481.
- Lamb M, Jorgensen W (1997) Computational approaches to molecular recognition. Curr Opin Chem Biol 1(4): 449-457.
- Cai W, Shao X, Maigret B (2002) Protein-ligand recognition using spherical harmonic molecular surfaces: Towards a fast and efficient filter for large virtual throughput screening. J Mol Graph Model 20(4): 313-328.
- Morris R, Najmanovich R, Kahraman A, Thornton J (2005) Real spherical harmonic expansion coefficients as 3D shape descriptors for protein binding pocket and ligand comparisons. Bioinformatics 21(10): 2347-2355.
- Kahraman A, Morris R, Laskowski R, Thornton J (2007) Shape variation in protein binding pockets and their ligands. J Mol Biol 368(1): 283-301.
- Perozzo R, Kuo M, Valiyaveettil J, Bittman R, Jacobs W, et al. (2002) Structural elucidation of the specificity of the antibacterial agent triclosan for malarial enoyl acyl carrier protein reductase. J Biol Chem 277(15): 13106-13114.
- Oliveira J, Pereira J, Canduri F, Rodrigues N, de Souza ON, et al. (2006) Crystallographic and pre-steady-state kinetics studies on binding of NADH to wild-type and isoniazid-resistant enoyl-ACP (CoA) reductase enzymes from Mycobacterium tuberculosis. J Mol Biol 359(3): 646-666.
- Schulze-Gahmen U, De Bondt HL, Kim SH (1996) High-resolution crystal structures of human cyclin-dependent kinase 2 with and without ATP: Bound waters and natural ligand as guides for inhibitor design. J Med Chem 39(23): 4540-4546.
- Chrencik J, Staker B, Burgin A, Pourquier P, Pommier Y, et al. (2004) Mechanisms of camptothecin resistance by human topoisomerase I mutations. J Mol Biol 339(4): 773-784.
- Timmers L, Caceres R, Vivan A, Gava L, Dias R, et al. (2008) Structural studies of human purine nucleoside phosphorylase: towards a new specific empirical scoring function. Arch Biochem Biophys 479(1): 28-38.
- Kuntz I, Blaney J, Oatley S, Langridge R, Ferrin T (1982) A geometric approach to macromolecule-ligand interactions. J Mol Biol 161(2): 269-288.
- Ewing T, Makino S, Skillman A, Kuntz I (2001) DOCK 4.0: Search strategies for automated molecular docking of flexible molecule databases. J Comput Aided Mol Des 15(5): 411-428.
- Lewis RA, Pickett SD, Clark DE (2009) Computer-Aided Molecular Diversity Analysis and Combinatorial Library. Rev Comput Chem 16: 1.
- Kramer B, Rarey M, Lengauer T (1999) Evaluation of the FLEXX incremental construction algorithm for protein-ligand docking. Proteins 37(2): 228-241.
- Van Der Spoel D, Lindahl E, Hess B, Groenhof G, Mark A, et al. (2005) GROMACS: Fast, flexible, and free. J Comput Chem 26(16): 1701-1718.
- Christen M, Hünenberger P, Bakowies D, Baron R, Bürgi R, et al. (2005) The GROMOS software for biomolecular simulation: GROMOS05. J Comput Chem 26(16): 1719-1751.
- Oostenbrink C, Villa A, Mark A, Van Gunsteren W (2004) A biomolecular force field based on the free enthalpy of hydration and solvation: the GROMOS force‐field parameter sets 53A5 and 53A6. J Comput Chem 25(13): 1656-1676.
- Liu M, Wang S (1999) MCDOCK: A Monte Carlo simulation approach to the molecular docking problem. J Comput Aided Mol Des 13(5): 435-451.
- Totrov M, Abagyan R (1997) Flexible protein-ligand docking by global energy optimization in internal coordinates. Proteins 29(S1): 215-220.
- Kirkpatrick S, Gelatt C, Vecchi M (1983) Optimization by simulated annealing. Science 220(4598): 671-680.
- Goodsell D, Olson A (1990) Automated docking of substrates to proteins by simulated annealing. Proteins 8(3): 195-202.
- Morris G, Goodsell D, Halliday R, Huey R, Hart W, et al. (1998) Automated docking using a Lamarckian genetic algorithm and an empirical binding free energy function. J Comput Chem 19(14): 1639-1662.
- Brooijmans N, Kuntz I (2003) Molecular recognition and docking algorithms. Annu Rev Biophys Biomol Struct 32(1): 335-373.
- Glover F, Laguna M (1993) Tabu Search. In: Reeves CR ed, and Modern Heuristic Techniques for Combinatorial Problems.
- Cho A, Rinaldo D (2009) Extension of QM/MM docking and its applications to metalloproteins. J Comput Chem 30: 2609-2616.
- Kitchen DB, Decornez H, Furr JR, Bajorath J (2004) Docking and scoring in virtual screening for drug discovery: Methods and applications. Nat Rev Drug Discov 3(11): 935-949.
- Kollman P (1993) Free energy calculations: Applications to chemical and biochemical phenomena. Chem Rev 93(7): 2395-2417.
- Åqvist J, Luzhkov VB, Brandsdal BO (2002) Ligand binding affinities from MD simulations. Acc Chem Res 35(6): 358-365.
- Carlson HA, Jorgensen WL (1995) An extended linear response method for determining free energies of hydration. J Chem Phys 99(26): 10667-10673.
- Verdonk M, Cole J, Hartshorn M, Murray C, Taylor R (2003) Improved protein-ligand docking using GOLD. Proteins 52(4): 609-623.
- Bohm H (1998) Prediction of binding constants of protein ligands: A fast method for the prioritization of hits obtained from de novo design or 3D database search programs. J Comput Aided Mol Des 12(4): 309-323.
- Gehlhaar D, Verkhivker G, Rejto P, Sherman C, Fogel D, et al. (1995) Molecular recognition of the inhibitor AG-1343 by HIV-1 protease: Conformationally flexible docking by evolutionary programming. Chem Biol 2(5): 317-324.
- Verkhivker G, Bouzida D, Gehlhaar D, Rejto P, Arthurs S, et al. (2000) Deciphering common failures in molecular docking of ligand protein complexes. J Comput Aided Mol Des 14(8): 731-751.
- Jain A (1996) Scoring noncovalent protein-ligand interactions: A continuous differentiable function tuned to computer binding affinities. J Comput Aided Mol Des 10(5): 427-440.
- Head R, Smythe M, Oprea T, Waller C, Green S, et al. (1996) VALIDATE: A New Method for the Receptor-Based Prediction of Binding Affinities of Novel Ligands. J Am Chem Soc 118: 3959-3969.
- Bohm H (1992) LUDI: Rule-based automatic design of new substituents for enzyme inhibitor leads. J Comput Aided Mol Des 6(6): 593-606.
- Eldridge M, Murray C, Auton T, Paolini G, Mee R (1997) Empirical scoring functions: I. The development of a fast-empirical scoring function to estimate the binding affinity of ligands in receptor complexes. J Comput Aided Mol Des 11(5): 425-445.
- Muegge I, Martin Y (1999) A general and fast scoring function for protein-ligand interactions: A simplified potential approach. J Med Chem 42(5): 791-804.
- Mitchell J, Laskowski R, Alex A, Thornton J (1999) Bleep-potential of mean force describing protein-ligand interactions: I. generating potential. J. Comput. Chem 20(11): 1165-1176.
- Ishchenko A, Shakhnovich E (2002) Small Molecule Growth 2001 (SMoG2001): An improved knowledge-based scoring function for protein-ligand interactions. J Med Chem 45(13): 2770-2780.
- Feher M, Deretey E, Roy S (2003) BHB: A simple knowledge-based scoring function to improve the efficiency of database screening. J Chem Inf Comput Sci 43(4): 1316-1327.
- Verkhivker G, Appelt K, Freer S, Villafranca J (1995) Empirical free energy calculations of ligand protein crystallographic complexes. I. Knowledge-based ligand-protein interaction potentials applied to the prediction of human immunodeficiency virus 1 protease binding affinity. Protein Eng 8(7): 677-691.
- Wallqvist A, Jernigan R, Covell D (1995) A preference based free-energy parameterization of enzyme-inhibitor binding. Applications to HIV-1-protease inhibitor design. Protein Sci 4(9): 1881-1903.
- Gohlke H, Hendlich M, Klebe G (2000) Knowledge-based scoring function to predict protein-ligand interactions. J Mol Biol 295(2): 337-356.
- DeWitte R, Shakhnovich E (1996) SMoG: de Novo Design Method Based on Simple, Fast, and Accurate Free Energy Estimates. Methodology and Supporting Evidence. J Am Chem Soc 118: 11733-11744.
- Charifson P, Corkery J, Murcko M, Walters W (1999) Consensus scoring: A method for obtaining improved hit rates from docking databases of three-dimensional structures into proteins. J Med Chem 42(25): 5100-5109.
- Feher M (2006) Consensus scoring for protein-ligand interactions. Drug Discov Today 11: 421-428.
- Clark R, Strizhev A, Leonard J, Blake J, Matthew J (2020) Consensus scoring for ligand/protein interactions. J Mol Graph Model 20(4): 281-295.
QUICK LINKS
- SUBMIT MANUSCRIPT
- RECOMMEND THE JOURNAL
-
SUBSCRIBE FOR ALERTS
RELATED JOURNALS
- Journal of Cancer Science and Treatment (ISSN:2641-7472)
- Journal of Psychiatry and Psychology Research (ISSN:2640-6136)
- Journal of Neurosurgery Imaging and Techniques (ISSN:2473-1943)
- Archive of Obstetrics Gynecology and Reproductive Medicine (ISSN:2640-2297)
- Journal of Pathology and Toxicology Research
- International Journal of Radiography Imaging & Radiation Therapy (ISSN:2642-0392)
- Journal of Blood Transfusions and Diseases (ISSN:2641-4023)